How Should I Manage X-ray Tomography Data? – The Danger of Relying on Hard Drives and How to Avoid Costly Problems
May 1, 2024
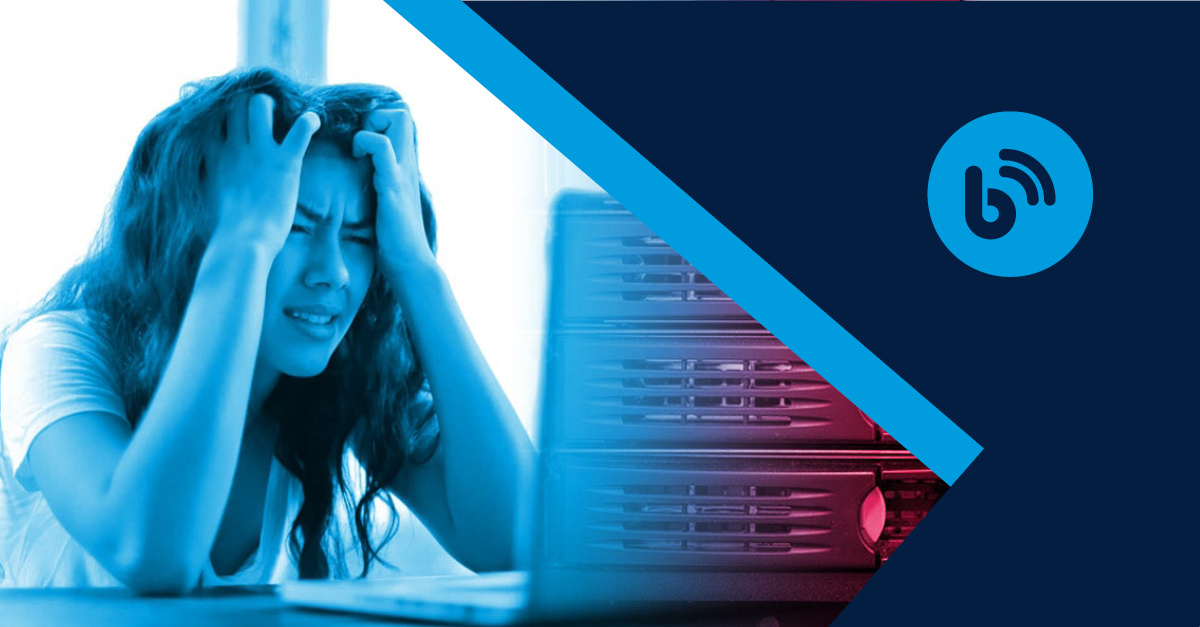
Introduction by: Aya Takase | Director of X-ray Imaging at Rigaku
Do you have a stack of external hard drives with your CT (computed tomography) data sitting in your office staring at you? Do you have nightmares about these drives dying and losing all the data you poured your blood, sweat, and tears into? Hard drives are not the only way to store large files like CT data. Actually, it is a pretty risky one. It is not just the storage hardware we need to consider. How you manage the data is equally important for the long-term success of a CT lab. In this article, our guest author, Dr. Shawn Zhang from DigiM Solution LLC, will show you the potential consequences of lack of data management and relying on a stack of hard drives. He will explain a better way to manage and store your CT data.
Prologue
Like it or not, we need a manager in pretty much every aspect of our civilized life. We may call it a boss, an administrator, a teacher, a parent, a calendar, a shopping list, or simply a notebook. When we fire that manager, the function of the organization and structure won’t go away. We then have to take things into our own hands, well, more like our own memory. We need to REMEMBER.
Tomography imaging data particularly needs to be managed. In this article, we will introduce data management as an essential element to the effective use of X-ray tomography imaging data. We will discuss why such a solution can’t rely on the manual effort from Microscopists’ memory, and share our own experience on how such a solution can be designed.
- Not managing data properly? - A costly pitfall
- The Why - Why do we need a special tool
- The How - How should we manage data?
- The When - When should we start implementing data management?
- Final remarks - What should I do now?
1. Not managing data properly? - A costly pitfall
First of all, these three-dimensional (3D) data are large, ranging from a few hundred MB to hundreds of GB. Unlike what we can do with our smartphone pictures, pulling them out to have a quick look is not easy. Specialized (and potentially costly) visualization software is often needed just to look at them, and that is still not quick. After only a few months of collecting these large 3D data, the data access problem grows beyond just visualization. The hard drive internal to your computer fills up quickly. Raw data, metadata, image analysis and segmentation data, quantitative plot data, movies, and PowerPoint slides constitute an ecosystem of valuable assets. Not willing to delete any of them, you start moving the data to external hard drives. However, tracking down what has been done, by who, using what resources can quickly become overwhelming to remember and starting to test our patience. It is often too late when we realize that we have too much data to deal with. Staring at the hard drives stacked up over the years, we wish to have a plan from the beginning.
The cost of lack of management mounts up in several ways.
At the basic level, it starts consuming our time. When a Microscopist spends time searching for data, waiting for the data to be copied, ordering hard drives, loading a large data file into local computer memory to just take a quick look, their time is wasted instead of being spent on what a Microscopist does best – collecting images or analyzing them. While each of these tasks may only be a few minutes, if you are dealing with hundreds of such datasets, 5 minutes can rapidly turn into 5 hours, 5 days, or 5 weeks of wasted time.
Secondly, even if you had plenty of time, archiving, accessing, and utilizing the data accumulated over the years itself can be a major challenge. Recall the cardboard boxes in your basement from your last relocation several years ago? If they were not labeled nor sorted appropriately, chances are that they will be sitting there collecting dust for years. You might have valuable and useful things in there, but you are not going to use them because you can’t find them or you have forgotten about them. The same thing has HAPPENED and still is happening to a lot of our data. That cost is beyond just time, as the microscopy data was often collected on some very expensive instruments, by some very expensive Microscopists, and on some very valuable samples. While such data sitting in a hard-drive somewhere collecting dust is unsettling for a Microscopist, it is a very bad return on investment for the business leaders.
And don’t even get me started if you are serious about analyzing the data. The pain scale below depicts vividly the situation.
Figure 1: Imaging data and workflow management pain scale
Yes, when a new imaging lab is set up with shiny instruments and powerful computers, everybody is perfectly happy (Figure 1A). After some samples are prepared and imaged, the rollercoaster ride starts. The raw imaging data is reconstructed, processed, segmented, analyzed, modeled, and reported. Several software platforms are typically needed. After a lot of steps and most likely some hair loss, we completed our first full workflow. While slightly discomforting (1B), we did it, didn’t we? Admittedly, image segmentation was distressing (1C), and modeling was often intense (1D). But we have the first results!
After we’ve done it the 10th time, lack of data management starts to make our image analytics life utterly horrible (1E). Moving the data, storing the data, following which data has been processed with what, by who, when, etc… — just thinking about them gives us a headache and causes some more hair loss. On top of the data management issue is the resource management challenge, what do you do if your boss calls you asking for a few images from the project you worked on last week, but your powerful workstation is performing complicated segmentation for the next three hours, and you can’t access the data your boss needs before his 2 pm meeting? What could be even worse and more embarrassing is that you can’t find the data your boss is asking for.
Now let’s fast-forward — after the imaging and analysis has been done on hundreds of samples for three years, unimaginable and unspeakable frustration is not an understatement of your feeling. This is why we Microscopists have thin hair. Well, that’s my theory.
2. The Why - Why do we need a special tool?
Microscopists pride themselves on getting things organized and keeping an eye out for detail. It is not that we are lazy or incompetent. It is the sheer amount of data and complexity of analysis workflow that demands efficient tools with a data management design to spare the burden on human memory. All desktop software tools have some kind of “management” features, e.g., scripting, project file packaging, and batch processing. However, they are mostly designed to deal with a small number of datasets, files much smaller than CT data, or workflows limited to 1 or 2 steps. They are not up for the job, although most people don’t realize this at the beginning. Remember the imaging data pain scale? At the stage of Figure 1A, when everybody was happy, it rarely, if ever, occurred to anyone that data management was a problem. The lab managers said, “The Microscopists will take care of it,” and shrugged. When the image acquisition software, analysis software, or modeling software was designed, the developers’ sole focus was most likely on the UI, analysis capabilities, cool 3D visualization features, etc. When it came to data management and data archiving, “The Microscopists will take care of it,” the developers said and shrugged.
The Microscopists need help to keep their hair.
3. The How - How should we manage data?
The solution to data management starts with an architectural decision. The corporate IT group could provide a data vault. For example, putting some software tools and storage on Amazon Web Services (AWS) will enable remote access. However, such data storage service is too general and merely a band-aid solution to the problems the Microscopists are dealing with. A complete data management solution will need to include:
- A management architecture
- Scalability
- Accessibility
- An integrated ecosystem with backward compatibility, data security, and data/workflow audit
A management architecture
Data management should be considered from day 1 of your new imaging lab. Otherwise, you will be dealing with the dusty boxes in the basement later. I will show you an example in Figure 2. This system, DigiM I2STM, is a managed web browser interface, with thumbnails automatically generated to provide a visual catalogue of your data. The imaging data and related metadata are managed by a correlational database. Important auxiliary information such as date stamps, users, and notes are recorded concurrently (Figure 2A). When an analysis is conducted, all analysis steps, parameters used, and derived data are recorded and annotated. Wouldn’t it be great if the data analysis and data management are done in one tool? It is also possible to put long computational tasks in a queue while new tasks are prepared in parallel. Computational resources can be allocated and usage recorded. An email notification with hyperlinks to task results can be sent to the user automatically upon completion of the task (Figure 2B). Routine visualizations and previews can also be generated automatically (Figure 2C).
Everything, yes, I truly mean everything, should be indexed and searchable (Figure 2D). It is apparent that a file explorer can’t cut the deal.
Figure 2: Managed and searchable browser interface of DigiM I2STM as an example data management solution.
Designed to scale
Scalability should be considered for datasets, storage, users, and computational needs. The system should be able to adjust to the growing needs of a user group analyzing new samples every day, adding new Microscopists, and employing more computationally-taxing analysis techniques. We want a system that can handle the upscaling from dozens of datasets to hundreds of datasets while simultaneously allowing the number of users and required computing resources to grow. 10 datasets, 1TB of storage, 1 user, with 1 computing thread for machine learning segmentation might have been enough in 2005, but you might need a system that can handle 1000 datasets, 1000TB of storage, 20 users with 100 computing threads of machine learning, deep learning, and flow simulations in 2030. And you probably don’t want to have to switch from one data management system to another along the way.
Scaling is not just about technology. The budget, IT management, new user onboarding, and knowledge sharing should all be part of the scaling considerations. Figure 3 below shows an architecture that takes into account all the aforementioned considerations and can truly scale. The key? A nested client-server architecture where the user access, the computing needs, and storage needs are all abstracted away with each application programming interface (API) layer supporting a client-server design.
Figure 3: An architecture for scalability, using DigiM I2S as an illustration, endorsed by seasoned industrial microscopist leaders.
Accessibility
For tomography data that requires a lot of resources (money and time) to acquire, accessibility is as important as the acquisition itself.
Consideration of accessibility starts with the users. How do users other than the Microscopists access the data? What if your collaborators or your boss want to see your results? If it is only accessible via a dedicated workstation using a dedicated software sitting in a dedicated room, the data has poor accessibility.
A shared drive improves accessibility and works okay for a few 2D images. It is, however, ineffective for larger 2D images, tiled 2D images, and 3D tomography images. A shared network drive could work okay, too. A general user can see that the dataset is there in a shared folder, but he still can’t really access it to see the images, let alone see the analysis results, without special software to open it or a dedicated workstation to run the software.
Once software comes into the discussion, licensing considerations soon follow. The users of tomography data have different needs. Some just need to look at the data, while others need to do involved image segmentation and quantitative analyses. The accessibility for these different users should also be provided at different levels, which many commercial software licenses do not account for.
A cloud computing architecture such as DigiM I2STM offers one of the best solutions. A web browser interface can easily provide remote access and support multiple uses’ simultaneous accesses without additional hardware. Figure 4 shows an example of a mosaic field of view (MFV) SEM images (such as Thermo Fisher MAPS or Carl Zeiss Atlas). The stitched image has 32,000 x 27,000 pixels (Figure 4A), a total file size of 8GB. Opening such a large 2D image and navigating it is difficult for any software running on an average desktop or laptop. A browser interface, supported by a hierarchical data structure at different resolutions, provides the maximum accessibility by any users, from anywhere, at any time, using any fixed or mobile computing devices. Artificial Intelligence (AI)-based segmentation can be conducted and visualized in the same manner through the cloud (Figure 4B). Real-time browsing and zooming on MFV-SEM dataset through the web-based interface provides a correlation between EM details in 2D (Figure 4C) and X-Ray tomography data in 3D (Figure 4D), without leaving the browser interface, using simply your mobile device (Figure 4E).
Figure 4: Large field of view, stitched scanning electron microscopy images correlated with high resolution MicroCT volume: access, visualize, and segment correlative imaging data via DigiM I2STM without a computer.
An Integrated Ecosystem: backward compatibility, data security, and data/workflow audit
Now we know what a well-managed and accessible 3D X-ray tomography database looks like. But what we need to manage is not just the image data, the storage, and the users. AI algorithms and models, computational physics simulation tools, computing resources, plotting, and reporting are all part of the management scope. An ecosystem is needed for building, sharing, and enjoying these computational tools among many users with different needs.
What about legacy data? No worries. They can be migrated from your old hard drives into a new, searchable, and scalable system. A data curation module in DigiM I2STM not only automates the integration of your legacy data but also streamlines the flow of your specific data management needs.
The idea of using cloud computing might worry some of you about data security. We cannot take data security lightly when talking about file sharing, remote accessibility, and multiple user support. However, we should not quickly judge cloud computing as insecure. The cloud is a computing framework, no different from a desktop computer as a computing framework with its own security challenges. Thus, the implementation of data security should be an independent design consideration. There are many ways to achieve a secure system. For example, a browser-based cloud computing system can be deployed fully on-premises, which from a security standpoint is no different than a node-locked software installed on a dedicated workstation. Don’t let the security concerns make you shy away from cloud solutions. Instead, choose the data management system that works best for you and remember to have the data security as one of the required features.
Let’s also not forget about reproducibility. From our (as Microscopists) own purpose of revisiting a dataset or a workflow in the future, to meeting the requirements from heavily regulated industrial applications such as FDA review of pharmaceutical products, it is of paramount importance that the ecosystem supports persistence, data integrity audits, and workflow reproducibility.
4. The When - When should we start implementing data management?
If you continue to do what you always have been doing, you will get what you’ve always been getting. That is you are relying on a Microscopists’ memory. I am sure that you have excellent Microscopists, but they are only human. The amount of data they need to organize based on their memory and self-discipline will hit their limit, and you will start losing the value of your precious data and your precious hair. We’ve been there. So when should we start implementing data management? The answer is “Now.”
5. Final remarks - What should I do now?
I hope I didn’t scare you too much about your data management and hair loss. DigiM I2STM [1] is one of the very few solutions out there that takes all the aspects mentioned here into consideration. It was designed by Microscopists to save their fellow peers, and industry-proven to solve big real-world problems by the FDA [2], the pharmaceutical companies [3], the material companies [4], and the oil and gas companies [5].
If you are interested in implementing a good data management system, discover our DigiM I2STM platform on the DigiM website, where you can also find additional webinars, videos, and paper downloads. Throw your data management challenges at us. We may not impress you with our hair, but you will not be disappointed otherwise.
Acknowledgment
The author acknowledges editorial inputs from Mr. Josh Lomeo at DigiM, and Ms. Aya Takase and Ms. Jing Gao at Rigaku.
References
-
Zhang, A.P. Byrnes, J. Jankovic, J. Neilly. Management, Analysis, and Simulation of Micrographs with Cloud Computing, Microscopy Today 27 (2) (2019) 26-33. 10.1017/S1551929519000026.
-
Shen, Y. Wang, S. Zhang, B. Qin, Q. Bao, D. Burgess. Characterization of the Stability of PLGA Microspheres Using Image-based Key Performance Attributes and Release Prediction, Poster presented at CRS 2021 Virtual Annual Meeting, 2021 Jul 25-29. (FDA complex drug product characterization workshop coverage by Dr. Darby Kozak on Youtube)
-
Nagapudi, A. Zhu, D. Chang, J. Lomeo, K. Rajagopal, R. Hannoush, S. Zhang. Microstructure, quality, and release performance characterization of long-acting polymer implant formulations with X-ray microscopy and quantitative AI analytics, Journal of Pharmaceutical Sciences. (2021). 10.1016/j.xphs.2021.05.016
-
Jankovic, S. Zhang, A. Putz, M.S. Saha, D. Susac. Multiscale imaging and transport modeling for fuel cell electrodes, Journal of Materials Research 34 (4) (2019) 579-591. 10.1557/jmr.2018.458.
-
P. Byrnes, S. Zhang, L. Canter, M.D. Sonnenfeld. Application of Integrated Core and Multiscale 3-D Image Rock Physics to Characterize Porosity, Permeability, Capillary Pressure, and Two and Three-Phase Relative Permeability in the Codell Sandstone, Denver Basin, Colorado, Paper presented at: Unconventional Resources Technology Conference, 2018 Jul 23-25; Houston, TX. 10.15530/URTEC-2018-2901840.
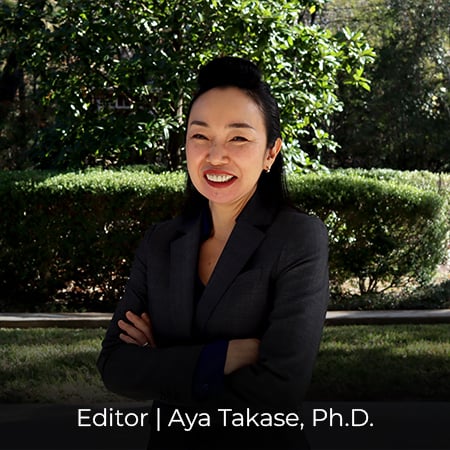
Subscribe to the X-ray CT Email Updates newsletter
Stay up to date with CT news and upcoming events and never miss an opportunity to learn new analysis techniques and improve your skills.
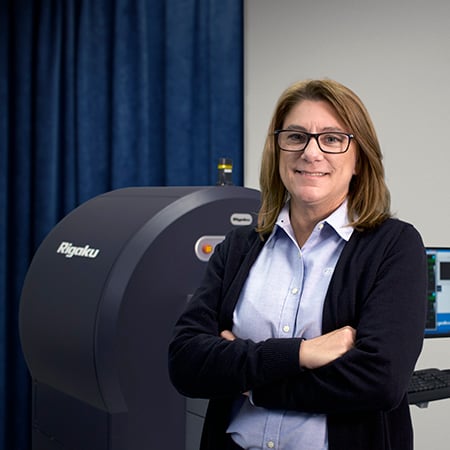
Contact Us
Whether you're interested in getting a quote, want a demo, need technical support, or simply have a question, we're here to help.