X-ray Reflectivity Analysis
How does AI help me?
This AI-powered module can suggest how to adjust your simulation model to improve the quality and accuracy of X-ray reflectivity (XRR) analysis.
A trained network can suggest what might be causing the discrepancy between the experimental and simulated profiles, such as a missing surface layer. Correcting the simulation model based on the suggestion can improve the accuracy of the XRR fitting.
How does AI learn to predict the cause of a discrepancy?
This module uses Vision Transformer, a neural network-based deep-learning algorithm. A neural network can be trained and learn what type of discrepancy between measured and simulated XRR profiles appears for the particular error in the model by using simulated data.
How does a neural network learn?
The neural network is modeled loosely on the human brain. The network architecture has input, hidden, and output layers with a number of “neurons.” The neurons are connected between the layers, and random weights and biases are assigned to these connections to set their “importance.” During training, the network processes input data (simulated and experimental XRR patterns) and predicts the output (possible error) using the initial weights and biases. Then, the prediction is compared to the ground truth. Depending on how close the output is to the ground truth, a “reward” or “penalty” is propagated through the network as “feedback.” By repeating this process, the network refines the weights and biases of the connections, which is equivalent to “learning” the relationship between the input and output.
Example 1
The simulation (blue line) is based on a model with two layers on a substrate. It does not reproduce the experimental profile (red line) well. The AI-assist model suggested the thickness of the first and second layers are reversed.
Example 2
The simulation (blue line) is based on a model with a superlattice on a substrate. It does not reproduce the experimental profile (red line) well. The number of fringes between the superlattice peaks does not match between the simulation and the experiment. The AI-assist model suggested the number of the superlattice period is incorrect.
Performance
Seven and nine types of suggestions are available for 1-2 layer models and superlattice models, respectively. The suggestion has been correct nine out of ten times in the cases tested so far.
Example 3
The simulation (blue line) is based on a model with a superlattice on a substrate. It does not reproduce the experimental profile (red line) well. The superlattice peaks stay narrow in the simulation, while the experimental data shows a broadening of the same peaks. The AI-assist model suggested the thickness (period) of the superlattice is not uniform.
Example 4
The simulation (blue line) is based on a model with two layers on a substrate. It does not reproduce the experimental profile (red line) well. The AI-assist model suggested a thin layer at the top surface is missing.
Adding the top surface layer to the model significantly improved the XRR fitting.
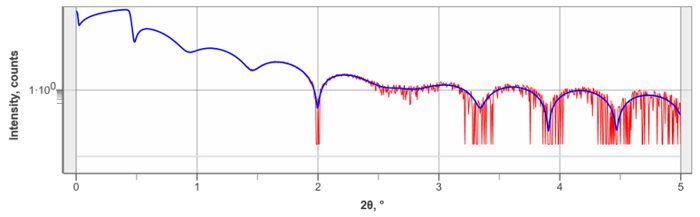
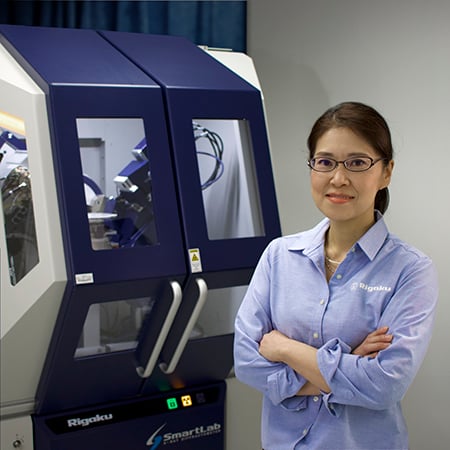
Contact Us
Whether you're interested in getting a quote, want a demo, need technical support, or simply have a question, we're here to help.